Project type: Design project at AI-powered Accounts Payable Solutions Company
Project length: 2 years
My role: Product Designer
Collaborators: Product managers, engineering team, customers, design manager
Project length: 2 years
My role: Product Designer
Collaborators: Product managers, engineering team, customers, design manager
Background: Smarter AP from day 1
*Company name has been fictionalized to white-label proprietary solutions.
When I joined Vexa.ai, it was already a leader in AI-driven invoice processing. While most solutions relied on OCR, Vexa.ai used AI to predict values based on invoice documents and past records from the same vendor, proving its value to major accounting firms in the U.S. and Europe.
With this foundation, the next move was expanding into the U.S. enterprise market, where companies process high invoice volumes daily. To meet enterprise needs, the company focused on enhancing its features—starting with purchase order (PO) matching, a key part of the accounts payable (AP) process.
The Challenge: Fixing PO matching pain points
Before paying an invoice, businesses often check it against a purchase order (PO)—a document that lists what was ordered, the quantity, and the agreed price. PO matching is the process of making sure these details line up. But this task is often slow and tedious, requiring a manual "stare and compare" to catch discrepancies. AI automation offered a major opportunity—intelligently matching invoices to POs while flagging any issues for human review.
As we researched PO matching, we quickly saw how complex it could be. There are different levels of matching: 2-way matching checks if the price and quantity on the invoice match the PO. 3-way adds another step by including a goods receipt to confirm the order was received. 4-way goes even further by adding an inspection to ensure the items meet quality standards.
On top of that, different types of POs require flexibility. For example, blanket POs cover recurring purchases over time, meaning multiple invoices might be linked to a single PO. Our system needed to track remaining balances, prevent overbilling, and ensure accurate matching—all without making the process more complicated for users.
This variability posed a design challenge—how to create an intuitive system that adapts to different matching rules without overwhelming users. While AI handled the complexity, our goal was a seamless, efficient, and user-friendly experience within existing workflows.
Impact: Why speed matters
Speeding up PO matching helps businesses process invoices faster, reduce errors, and avoid payment delays. It improves cash flow, unlocks early payment discounts, and prevents bottlenecks as companies scale. Automation saves time, cuts costs, and ensures smoother financial operations.
Existing System: Where we started
Here’s a quick overview of the invoice module. Invoices can be forwarded via email or manually uploaded into the system, where key data is automatically extracted. The document viewer on the right displays the invoice for easy reference. AI confidence scores are visually represented with color-coded lines: red for low confidence, orange for medium confidence, and green for high confidence. AP and invoice processing specialists focus on reviewing and correcting low-confidence data to ensure accuracy.
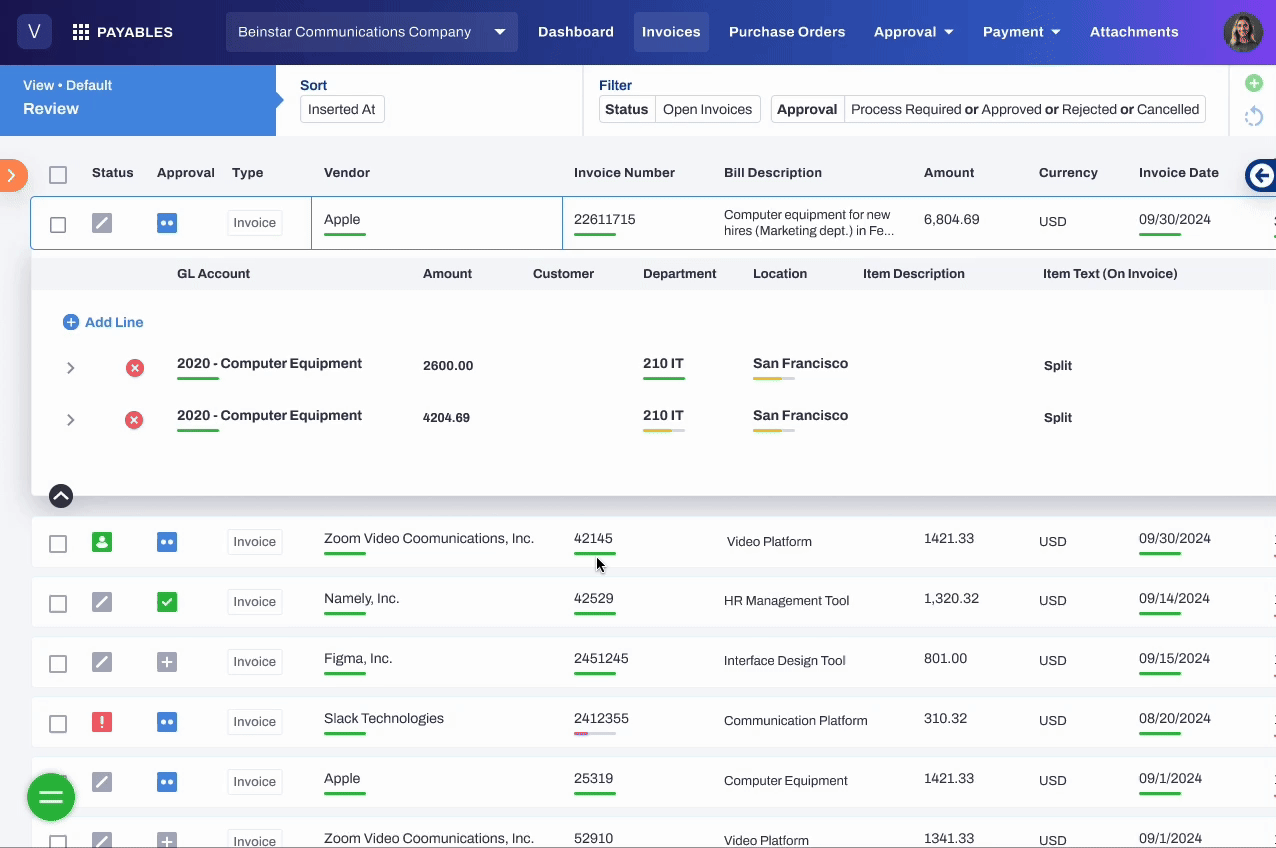
Design Strategies for Efficient PO Matching
1. Integrate the new workflow seamlessly.
a. For AP specialists, PO matching is a secondary task after verifying invoice data. To avoid overwhelming users, we introduced a "View PO" toggle, allowing them to review POs only after completing invoice validation.
b. To maintain consistency with the existing data table design, we added shaded columns to display matched PO data alongside invoice details, making comparisons easy.
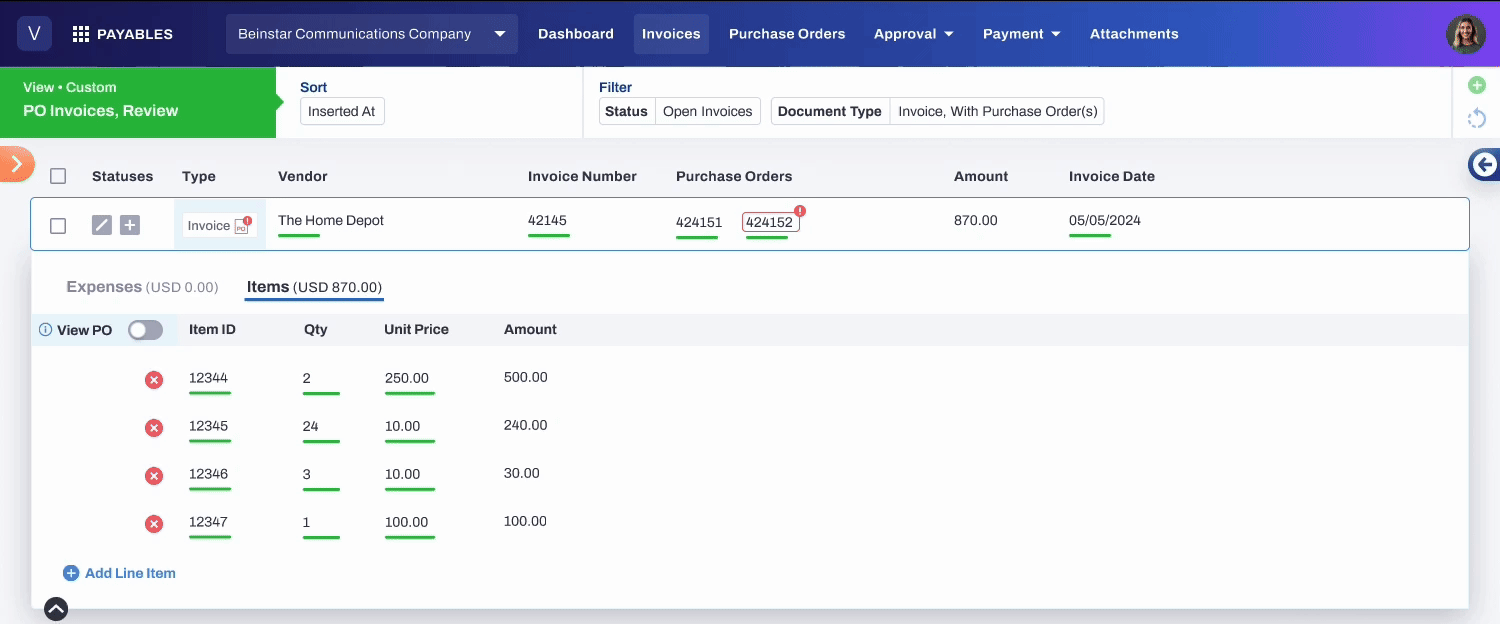
c. Once a discrepancy was detected, we also designed a seamless approval workflow for AP specialists to request approvals from the right person, often the PO requester. To support this, we created a place for users to define approval flows for discrepancies. Since our system already handled invoice approvals, we were able to adapt the existing design patterns—though engineering faced additional complexities.
A new addition to the workflow was tolerance levels, allowing users to define acceptable deviations before triggering an approval. For example, if an invoice amount is within 5% over the original PO, the AP team could approve payment without involving procurement. Approvers were then notified when discrepancies required action, and this workflow closely followed the existing invoice approval experience, ensuring a familiar and intuitive process.
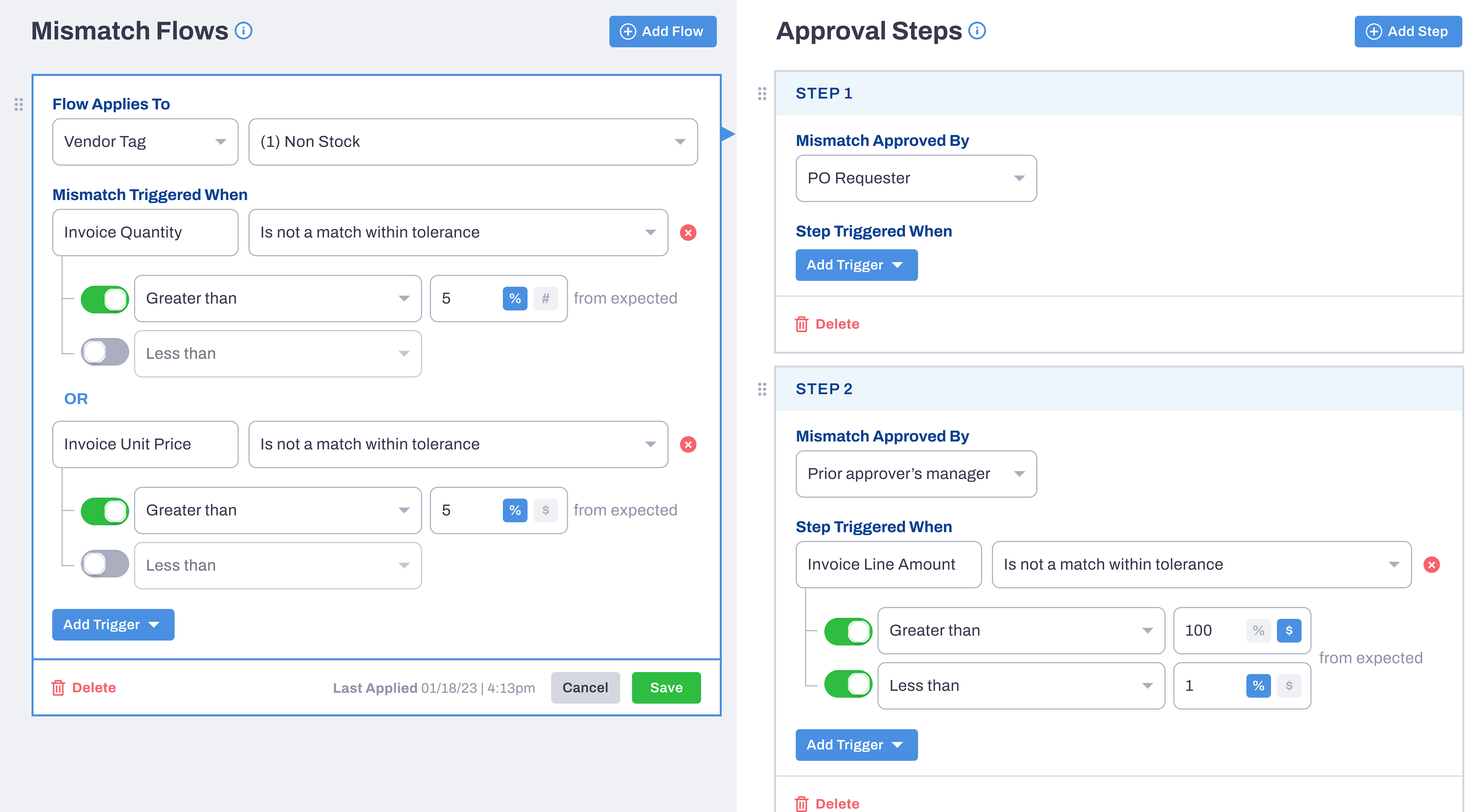
Admin page to define PO mismatch approval flows
2. Utilize the existing design patterns within the system.
Our AI does the heavy lifting of PO matching, but a key design challenge was ensuring that users could quickly spot and resolve issues. We needed to highlight cases requiring attention.
a. We added icons to the existing 'Invoice Type' tag to indicate whether an invoice was tied to a PO and provide additional information at a glance:
● Successfully matched (green): no human review needed
● Approval in progress (blue): waiting for someone's review
● PO matching issues (red): requires attention because AI found mismtaches between the invoice and PO.
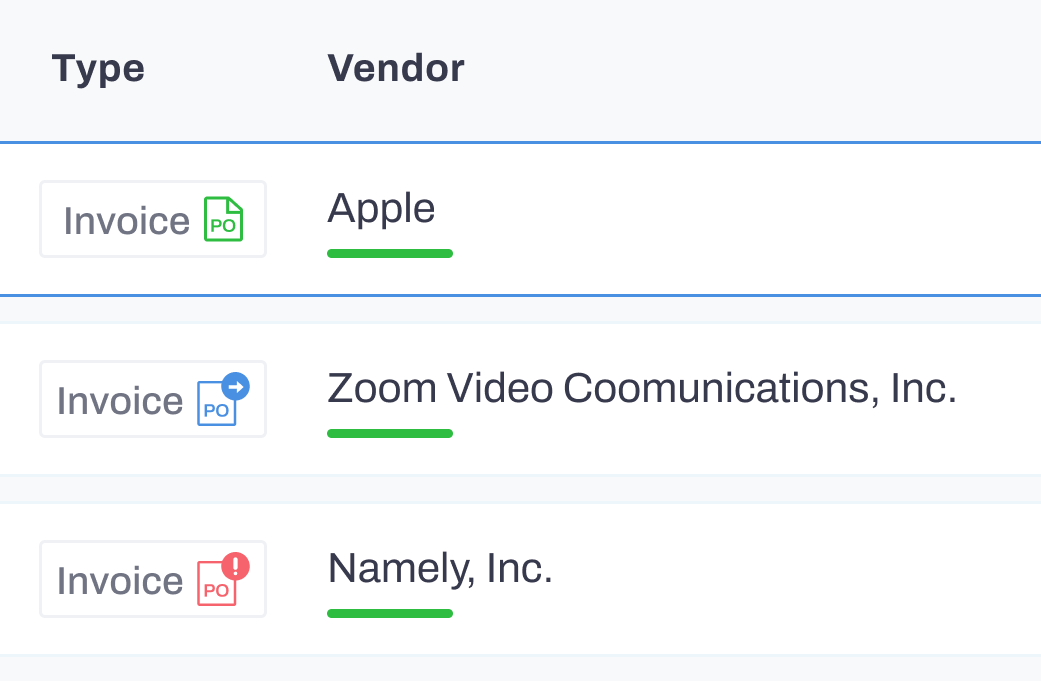
b. Since we already highlight incorrect values (e.g., Due Date errors) in red with an exclamation mark, we applied the same design for PO-related issues or missing information.
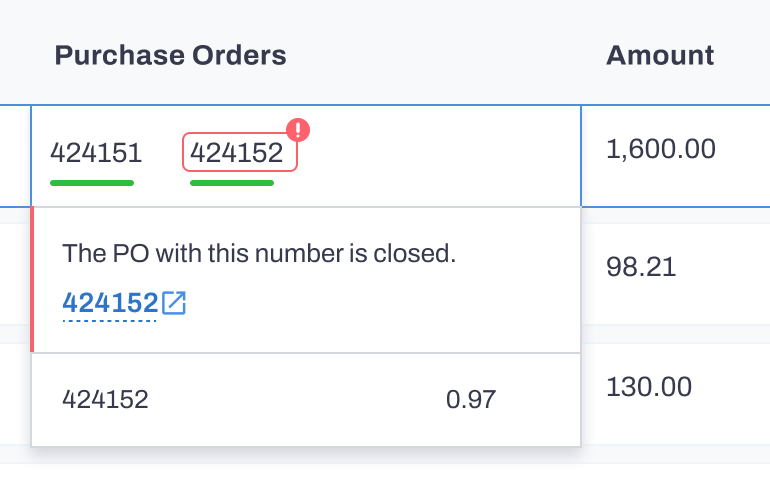
c. While the green line typically indicates high confidence from AI, we introduced a double line to signify even greater AI involvement. The double line indicates that, although the invoice lacks a PO number, AI has identified a likely associated PO based on a matched line item.
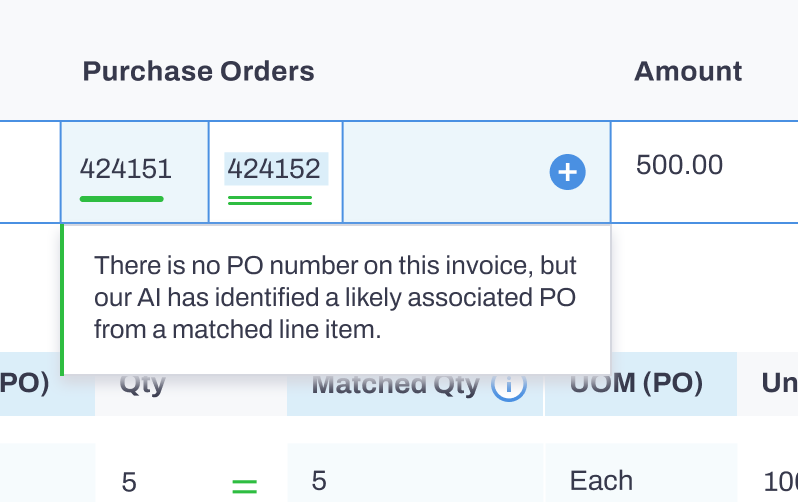
d. Purchase Order numbers were made clickable, opening in a new tab for detailed review. Additionally, we implemented a similar toggle on the Purchase Order page, allowing users to easily compare the items in the PO with existing invoices in the system.
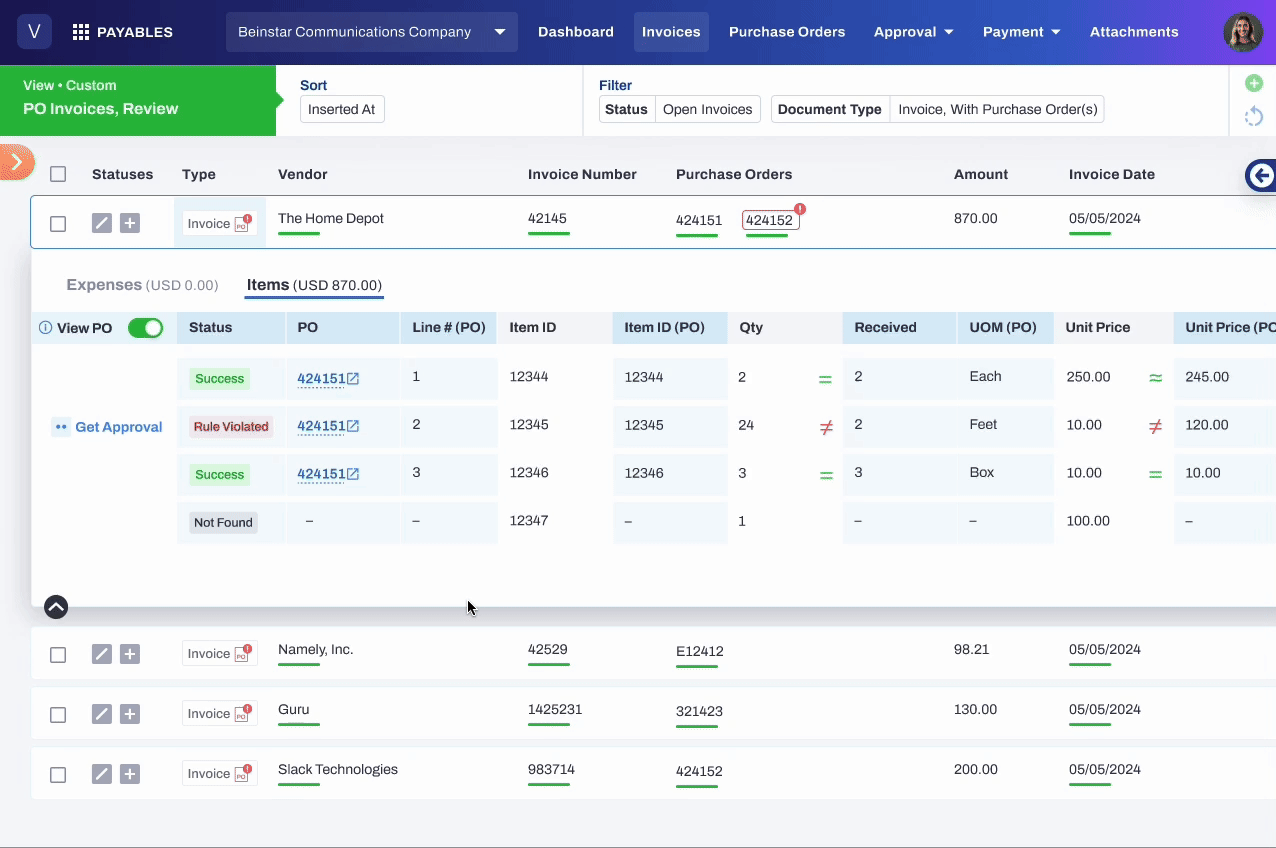
3. Consider every detail with thoughtful design.
a. We introduced symbols between cells for quick review:
= Exact match
≠ Mismatch
≈ Within approved tolerance
Hovering over cells further gives more details about the discrepancy between the invoice and PO values.
Hovering over cells further gives more details about the discrepancy between the invoice and PO values.
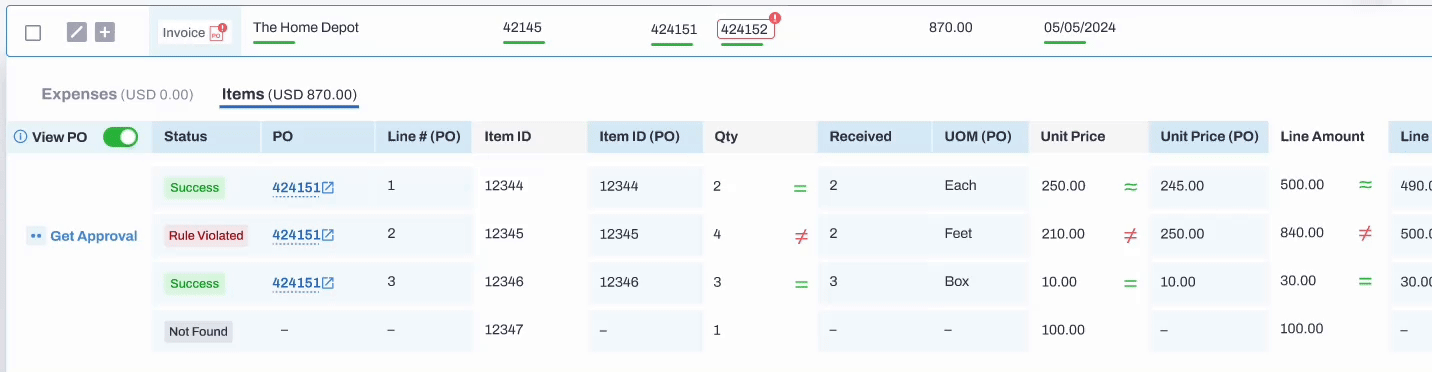
Symbols between invoice & PO values
b. To streamline issue resolution, we introduced a new popover-style modal that allows users to easily initiate the approval process when a discrepancy is detected. While this was a new style of component, it felt warranted, as it is embedded within the screen, allowing users to stay in context and continue seamlessly with their workflow.
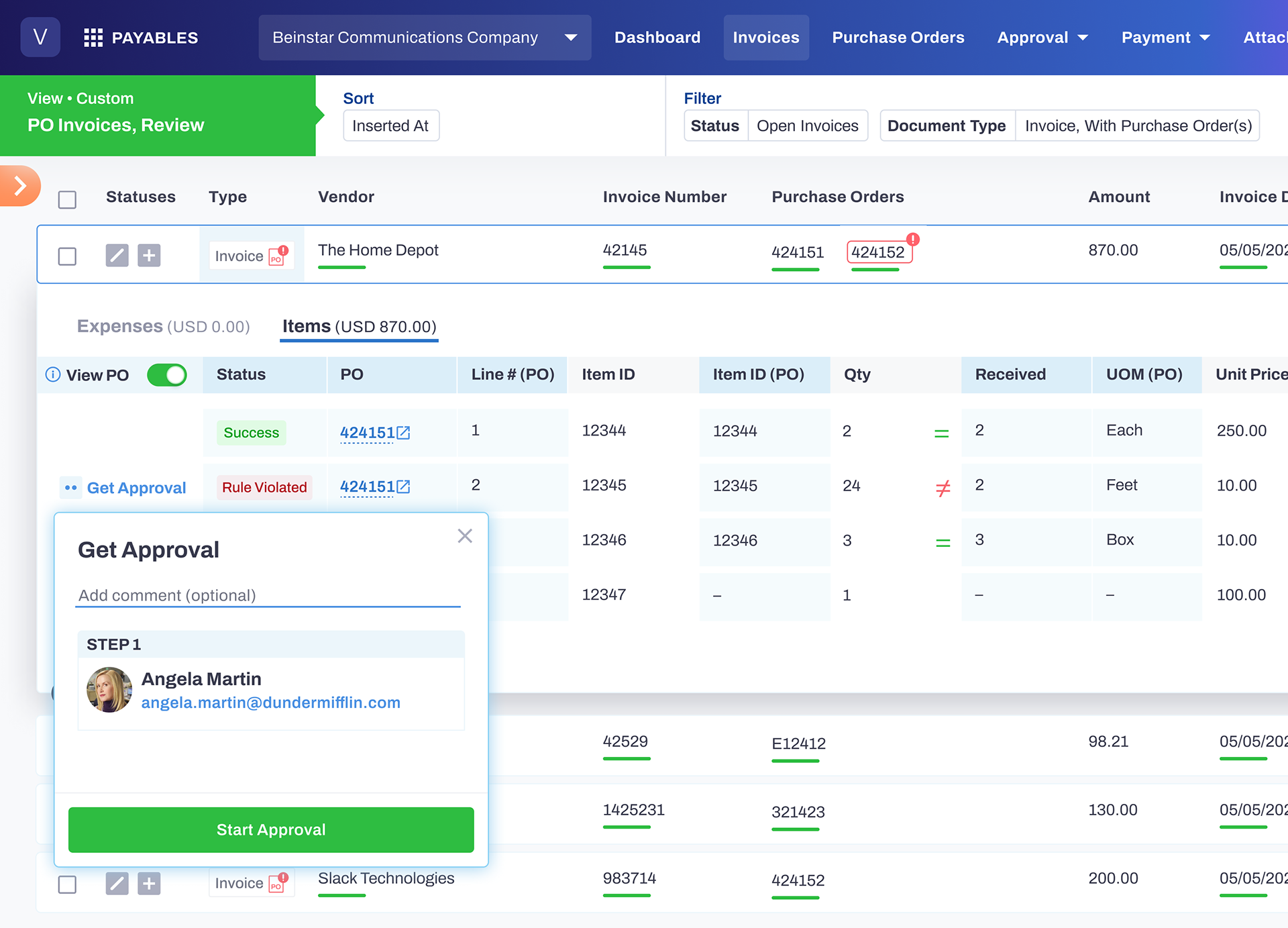
A modal to start the approval process
Final Thoughts
These designs are just a glimpse into a complex, multi-year project. Early on, we faced challenges such as a slow development ramp-up and the uncertainty of working with only one beta customer, limiting our exposure to edge cases. To mitigate these risks, I created Figma prototypes that our sales engineers used to gather feedback and validate concepts. Additionally, I leveraged research via ChatGPT and other sources to deepen my knowledge and become more of a subject matter expert. As the project evolved, onboarding more customers gave us deeper insights into blanket PO use cases and different levels of PO matching, allowing us to refine and strengthen the PO matching module into a more robust and comprehensive solution.
As of 2025, a year after the PO Matching module's release, 9 customers were actively using it, offering valuable feedback that’s been key to continuous improvements. In response to varying customer needs, the product team has also introduced a simplified version of the module, focusing on document-level rather than line-level matching, making it more accessible to customers with simpler matching requirements. This module continues to be a key product offering at Vic.ai, evolving to meet diverse customer needs and drive long-term value.